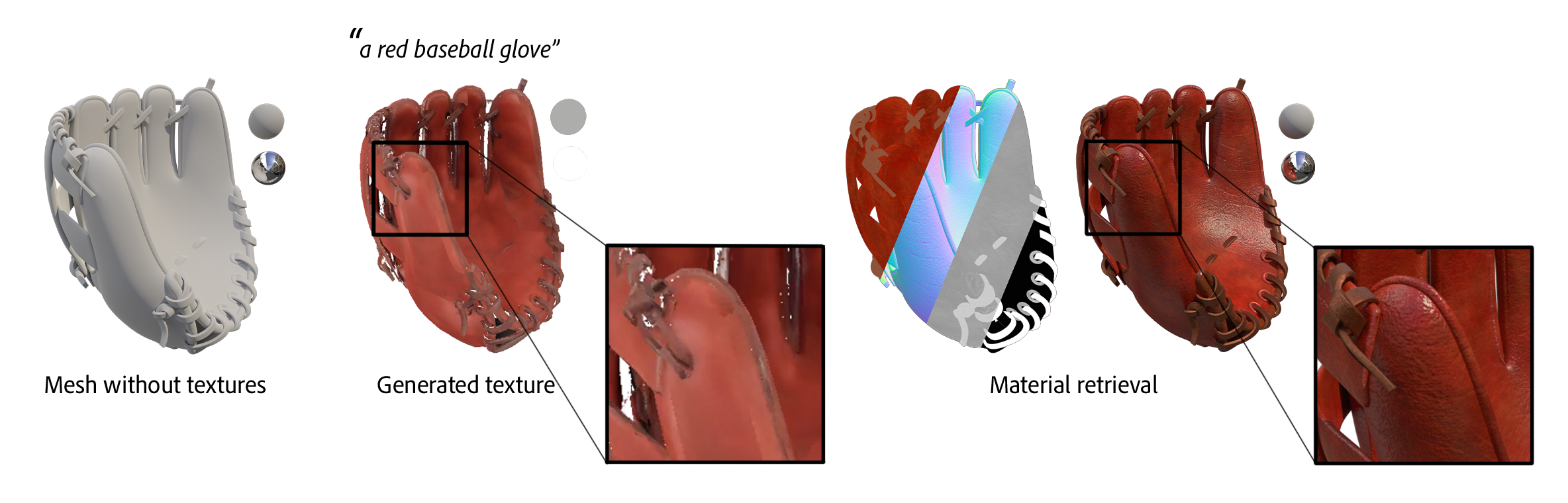
Abstract
We present MatAtlas, a method for consistent text-guided 3D model texturing. Following recent progress we leverage a large scale text-to-image generation model (e.g., Stable Diffusion) as a prior to texture a 3D model. We carefully design an RGB texturing pipeline that leverages a grid pattern diffusion, driven by depth and edges. By proposing a multi-step texture refinement process, we significantly improve the quality and 3D consistency of the texturing output. To further address the problem of baked-in lighting, we move beyond RGB colors and pursue assigning parametric materials to the assets. Given the high-quality initial RGB texture, we propose a novel material retrieval method capitalized on Large Language Models (LLM), enabling editabiliy and relightability. We evaluate our method on a wide variety of geometries and show that our method significantly outperform prior arts. We also analyze the role of each component through a detailed ablation study.
![]() |
![]() |
![]() |
![]() |
---|
![]() |
![]() |
![]() |
![]() |
---|
![]() |
![]() |
![]() |
![]() |
---|
Material assignment results on Source 3D Assets
For each of the following 3D models from the Souce 3D Assets dataset, we show the generated texture, the material part segmentation, and the result obtained by retreiving materials for each part. In the last column, for each material group provided by the asset, we provide a link to the retreived Substance material.
generated texture
|
segmentation
|
material assignment
|
---|
generated texture
|
segmentation
|
material assignment
|
---|
generated texture
|
segmentation
|
material assignment
|
---|
generated texture
|
segmentation
|
material assignment
|
---|
generated texture
|
segmentation
|
material assignment
|
---|
generated texture
|
segmentation
|
material assignment
|
---|
generated texture
|
segmentation
|
material assignment
|
---|
generated texture
|
segmentation
|
material assignment
|
---|
generated texture
|
segmentation
|
material assignment
|
---|
generated texture
|
segmentation
|
material assignment
|
---|
generated texture
|
segmentation
|
material assignment
|
---|
generated texture
|
segmentation
|
material assignment
|
---|
generated texture
|
segmentation
|
material assignment
|
---|
generated texture
|
segmentation
|
material assignment
|
---|
Material assignment results on Objaverse models
For each of the following 3D models from the Objaverse dataset, we show the generated texture, the material part segmentation, and the result obtained by retreiving materials for each part.
generated texture
|
segmentation
|
material assignment
|
---|
generated texture
|
segmentation
|
material assignment
|
---|
Comparison of textured results with state-of-the-art methods on Objaverse
We provide additional comparisons between our texture generation method and baselines.
ours
|
Text2Tex
|
TEXTure
|
---|
ours
|
Text2Tex
|
TEXTure
|
---|
ours
|
Text2Tex
|
TEXTure
|
---|
ours
|
Text2Tex
|
TEXTure
|
---|
ours
|
Text2Tex
|
TEXTure
|
---|
ours
|
Text2Tex
|
TEXTure
|
---|
ours
|
Text2Tex
|
TEXTure
|
---|
ours
|
Text2Tex
|
TEXTure
|
---|